How do winemakers at E. & J. Gallo use 25% less water to grow better grapes? With IBM Watson’s analysis of weather reports and satellite imagery, winemakers can increase irrigation efficiency and improve grape quality. Only with Watson IoT + IBM Cloud. Learn more: https://ibm.co/2nM25Ac
Category: Predictive
The complete set of conditions throughout a growth cycle can be thought of as a climate recipe, and each recipe produces unique phenotypic expressions, or physical qualities in different plants.
Building a Recipe
scalability & infrastructure
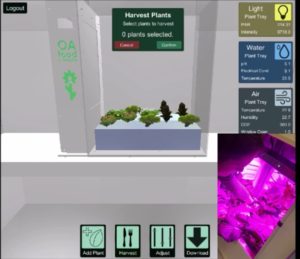
“Inside of a Food Computer, climate variables such as
- carbon dioxide,
- air temperature,
- humidity,
- dissolved oxygen,
- potential hydrogen,
- electrical conductivity,
- root-zone temperature, and more can be controlled and monitored.
Usage specifications such as
- operational energy,
- water use,
- and mineral consumption can also be monitored and adjusted through, electrical meters, flow sensors, and controllable chemical dosers throughout the growth period.
The complete set of conditions throughout a growth cycle can be thought of as a climate recipe, and each recipe produces unique phenotypic expressions, or physical qualities in different plants.”
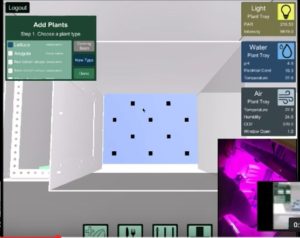
“Plants grown under different conditions may vary in color, size, texture growth rate, yield, flavor, and nutrient density.
Food computers could be used program biotic and abiotic stresses, such as an induced drought, to create desired plant-based expressions.
It would even be possible to monitor existing natural climates and program them into downloadable recipes that could be shared around the globe.
With the creation of climate recipes, food computer users can import successful climates that have been created, tested, and perfected by other users.
The recipes can be customized and optimized for different taste or yield preferences and for various food production needs.” – Open Ag Climate Recipes – Massachusetts Institute of Technology
________________________________________________________________________
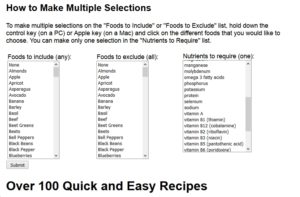
“Beyond food, we are now applying this research in other industries. A system that can model human preferences and generate new ideas has many applications outside of food and the opportunity to transform customer experience.
Truly superior customer experiences are based on perception—appealing taste,
appearance and design, to name a few—and represent a major differentiator in a variety of industries, including retail, consumer goods, hospitality and travel.
As companies race to bring new products to mark computational creativity can accelerate how quickly they can bring products to market, reduce the cost of R&D, while helping them design what differentiating features should be prioritized for competitive edge.” – Cognitive Cooking Fact Sheet – IBM
Cognitive Computation
The biggest machine thinkers in the room
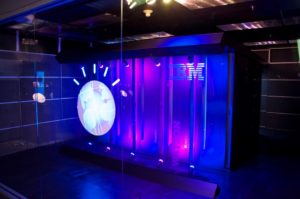
1.IBM Watson: IBM Watson, leverages deep content analysis and evidence based reasoning to accelerate and improve decisions, reduce costs and optimize outcomes. …
2.Microsoft Cognitive Computers
3.HPE Haven OnDemand
4.Cisco Cognitive Threat Analytics
5.Google DeepMind
6.CustomerMatrix
7.SparkCognition
Predictive
automated greenhouse technology
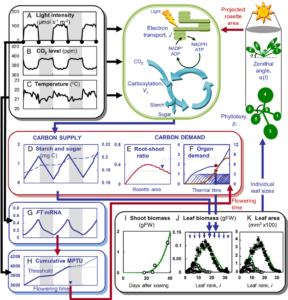
Being able to predict future growth with Tradeoff Analytics – “Trade-offs, by which we mean exchanges that occur as compromises, are ubiquitous when land is managed with multiple goals in mind. Trade-offs may become particularly acute when resources are constrained and when the goals of different stakeholders conflict (Giller et al. 2008).
In agriculture, trade-offs between output indicators may arise at all hierarchical levels,
- from the crop (such as grain vs. crop residue production),
- the animal (milk vs. meat production),
- the field (grain production vs. nitrate leaching and water quality),
- the farm (production of one crop vs. another),
- to the landscape and above (agricultural production vs. land for nature).
An individual farmer may face trade-offs between maximizing production in the short term and ensuring sustainable production in the long term. Within landscapes, trade-offs may arise between different individuals for competing uses of land…
Understanding the system dynamics that produce and change the nature of the trade-offs is central to achieving a sustainable and food secure future.” –Measurement Methods for environment–productivity trade-off analysis in agricultural systems
M.T. van Wijk3, h.J. Klapwijk1,2*, Todd S. Rosenstock4, Piet J.A. van Asten2, Philip K. Thornton5 and Ken E. Giller1